July 12, 2024|Industry Specific AI Updates
Explore AI’s energy challenge: from current constraints driving innovation to potential fusion breakthroughs. Discover how the quest for sustainable AI is shaping the future of technology and our planet.
.png/:/cr=t:0%25,l:0%25,w:100%25,h:100%25/rs=w:1280)
Introduction: The Hidden Cost of AI’s Power
Artificial Intelligence (AI) has driven technological innovation, revolutionizing industries from healthcare to finance. However, behind the scenes of this AI renaissance lies a growing concern: the enormous energy consumption required to fuel these advancements. As we stand on the brink of an AI-powered future, it’s crucial to understand how energy constraints are not just limiting progress but also shaping the very trajectory of AI development.
The Growing Energy Demand for AI
The computational demands of AI, particularly deep learning models, are staggering. To put this into perspective:
- Training a single large language model with 175 billion parameters can consume approximately 1,287 MWh of energy.
- This is equivalent to the annual electricity usage of about 121 average U.S. homes.
- To further illustrate, if this energy were used to power electric vehicles, it could drive a Tesla Model 3 for over 3.7 million miles – that’s more than 148 times around the Earth!
These figures highlight a critical challenge: as AI models grow more complex, their energy requirements are skyrocketing. This trend raises important questions about the sustainability of current AI development practices and underscores the need for more energy-efficient AI technologies.
Training vs. Inference Energy Consumption
Understanding the distinction between training and inference energy consumption is crucial.
To grasp the full picture of AI’s energy consumption, it’s essential to distinguish between two key processes:
- Training: The initial, energy-intensive phase where an AI model learns from vast datasets. This process can take weeks or even months for large models.
- Inference: The application of a trained model to make predictions or decisions. While less energy-intensive than training, the cumulative energy cost of inference can be significant due to its frequent use.
Environmental Impact: AI’s Carbon Footprint
The environmental implications of AI’s energy hunger are profound:
- Training a single large AI model can generate carbon emissions equivalent to the lifetime emissions of five cars.
- The AI industry’s carbon footprint is growing at an alarming rate, with some estimates suggesting it could account for up to 3.5% of global greenhouse gas emissions by 2030.
These statistics underscore the urgent need for sustainable solutions in AI development.
Challenges Posed by Energy Constraints
Energy constraints present several critical challenges to the AI industry:
- Computational Efficiency: The need for algorithms and hardware that can perform complex tasks with minimal energy consumption.
- Hardware Limitations: Current CPUs and GPUs struggle to meet the growing energy demands of AI models efficiently.
- Scalability Issues: As AI models increase in complexity, the energy required to train and deploy them grows exponentially.
- Innovation Bottlenecks: Limited power availability can lead to slower development cycles and restricted access to advanced AI capabilities.
Energy constraints pose several challenges to AI development. These challenges necessitate rethinking current AI development practices and exploring innovative solutions.
Innovative Solutions: Powering AI Sustainably
Researchers and tech companies are actively working on solutions to address these energy challenges:
1. Hardware Evolution
- Development of energy-efficient processors and specialized AI chips
- Exploration of alternative computing paradigms like quantum and neuromorphic computing
2. Algorithmic Efficiency
- Techniques such as model pruning and knowledge distillation to reduce model size without sacrificing performance
- Development of algorithms like DeepMind’s JEST, which significantly reduces energy consumption during training
3. Sustainable Infrastructure
- Shifting data centers to renewable energy sources
- Implementing smart cooling systems and energy management in AI facilities
4. Alternative Training Methods
- Transfer learning: Leveraging pre-trained models to reduce overall training time and energy
- Federated learning: Distributing training across multiple devices to optimize resource use
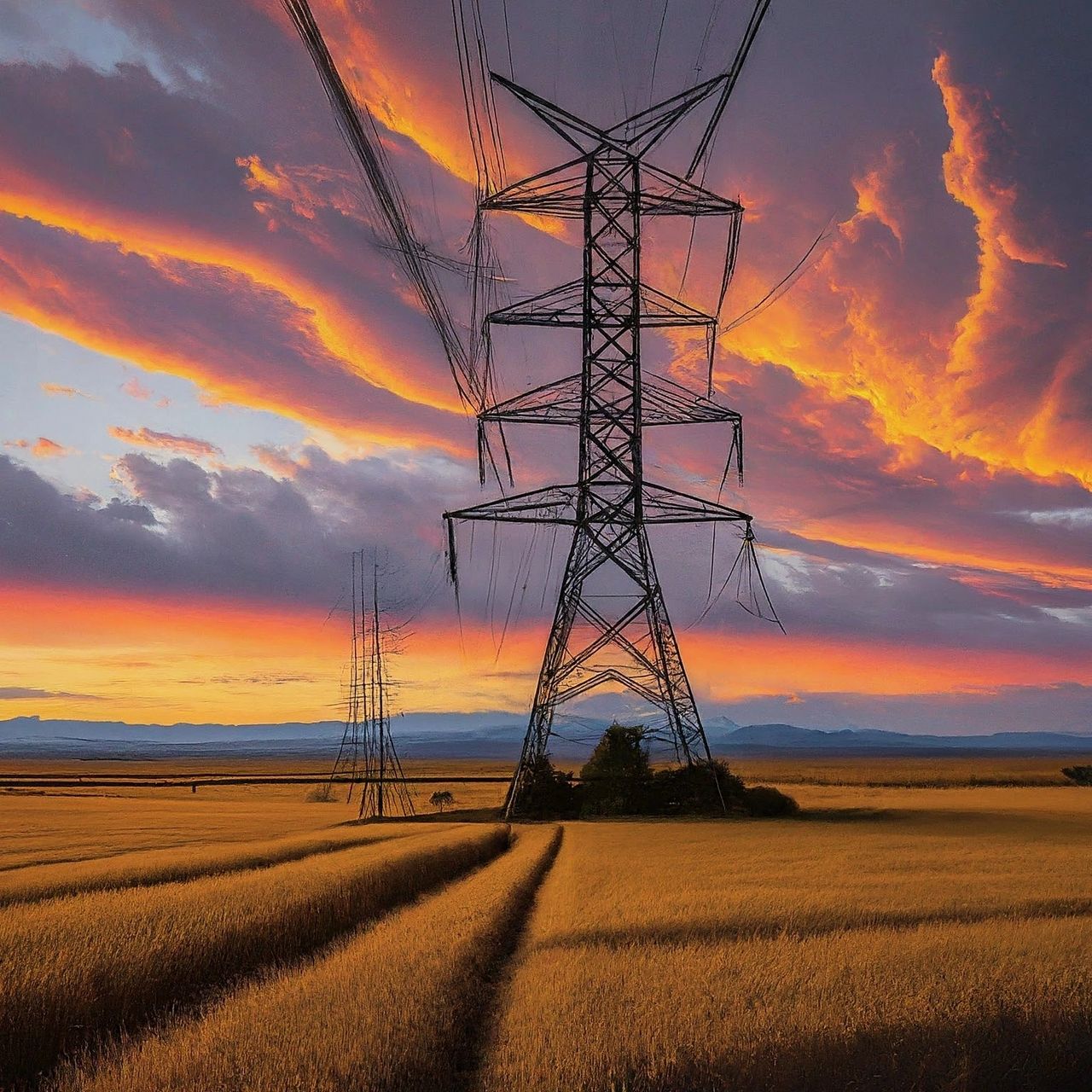
Sustainable Solutions for AI Training
The environmental impact of increased power consumption for AI is a growing concern. Here’s how we can address it:
- Harnessing Renewables: Shifting data centers and training facilities to renewable energy sources like solar, wind, or geothermal power can significantly reduce the environmental footprint.
- Cloud Optimization: Cloud computing platforms can be optimized for AI workloads by utilizing smarter resource allocation and leveraging idle computing power during off-peak hours. Imagine training models when everyone’s asleep!
- Alternative Training Methods: Techniques like transfer learning (leveraging pre-trained models) and federated learning (distributing training across devices) can reduce the overall power required for training specific AI models.
Energy Constraints as Catalysts for Innovation
While energy constraints pose significant challenges to AI development, they are also driving unprecedented innovation in the field. This “necessity is the mother of invention” scenario is pushing AI researchers and developers to think creatively and develop groundbreaking solutions:
- Algorithm Optimization: The need for energy efficiency is spurring the development of more sophisticated algorithms that can achieve better results with less computational power. This includes:
- Sparse neural networks that use fewer parameters
- Efficient attention mechanisms in transformer models
- Neural architecture search to find optimal, energy-efficient model structures
- Hardware Innovations: Energy limitations are accelerating the development of specialized AI hardware:
- Neuromorphic chips that mimic the energy efficiency of the human brain
- Photonic computing that uses light instead of electricity to process information
- 3D-stacked memory to reduce data movement and energy consumption
- Cloud and Edge Computing Synergy: The push for energy efficiency is leading to better integration of cloud and edge computing:
- Distributed AI systems that balance computation between energy-intensive data centers and low-power edge devices
- Adaptive AI models that can scale their complexity based on available energy resources
- Green AI Practices: Energy constraints are fostering a culture of sustainability in AI development:
- Carbon-aware training schedules that optimize for both performance and emissions
- Life cycle assessments for AI models to understand and minimize their long-term energy impact
These innovations not only address immediate energy concerns but also have the potential to make AI more accessible and deployable in a wider range of scenarios, from resource-constrained IoT devices to large-scale industrial applications.
Fusion Breakthroughs: A Game-Changer for AI?
Recent advancements in fusion energy research have sparked excitement in the AI community. Fusion, the process that powers the sun, promises virtually limitless clean energy. But what could this mean for AI development?
- Unlimited Training Potential: With fusion energy, the current limitations on model size and training duration could become obsolete. This could lead to:
- AI models of unprecedented scale and complexity
- Extended training periods for more sophisticated and accurate models
- Exploration of entirely new AI architectures that were previously impractical due to energy constraints
- Democratization of AI: Abundant, cheap energy could make high-performance AI more accessible:
- Reduced costs for AI research and development
- More organizations and individuals able to participate in cutting-edge AI projects
- Potential for AI-driven solutions to be deployed more widely in developing regions
- Environmental Benefits: Fusion-powered AI could significantly reduce the carbon footprint of the technology sector:
- Carbon-neutral AI training and inference at scale
- Potential for AI to be used more extensively in climate change mitigation efforts
- New Frontiers in AI Applications: With energy constraints removed, AI could be applied to solving even more complex problems:
- Advanced climate modeling and prediction
- More comprehensive simulations for drug discovery and materials science
- Large-scale optimization of global systems (e.g., supply chains, energy grids)
However, it’s important to note that viable fusion energy is still years away from commercial reality. In the meantime, the AI community must continue to focus on energy efficiency and explore other alternative energy sources.
Alternative Energy Sources Powering AI’s Future
While fusion represents a potential long-term solution, various alternative energy sources are already being harnessed to power AI development more sustainably:
- Solar and Wind Power:
- Many tech companies are investing in large-scale solar and wind farms to power their data centers.
- Example: Google’s data centers are matched with 100% renewable energy on an annual basis.
- Hydroelectric Power:
- Some AI companies are strategically locating their data centers near hydroelectric power sources.
- This provides a consistent and renewable energy supply for AI operations.
- Geothermal Energy:
- Countries like Iceland are leveraging their geothermal resources to attract AI and blockchain companies.
- Geothermal energy offers a stable, renewable power source for energy-intensive computations.
- Nuclear Energy:
- Despite controversies, some argue that nuclear power could provide the steady, high-volume energy needed for large-scale AI operations with minimal carbon emissions.
- Advanced nuclear technologies like small modular reactors (SMRs) could provide localized power for AI facilities.
- Hydrogen Fuel Cells:
- Some data centers are experimenting with hydrogen fuel cells as a backup power source.
- This technology could potentially be scaled to provide primary power for AI operations in the future.
- Biomass and Waste-to-Energy:
- These technologies could provide supplementary power for AI facilities while addressing waste management issues.
- Energy Storage Solutions:
- Advancements in battery technology and other energy storage methods are crucial for managing the intermittent nature of some renewable sources.
- This enables AI operations to run consistently even when using variable energy sources like solar or wind.
By diversifying energy sources and investing in renewable technologies, the AI industry can reduce its reliance on fossil fuels and create a more sustainable foundation for future growth. This shift not only addresses environmental concerns but also provides resilience against energy price volatility and supply disruptions.
Pioneers in Sustainable AI Practices
Several tech giants are leading the charge in promoting energy-efficient AI:
- Google: Optimizing data centers with AI-powered cooling systems and integrating renewable energy
- DeepMind: Developing energy-efficient AI models and algorithms
- IBM: Pioneering quantum computing research for AI applications. Quantum computers could perform complex calculations with a fraction of the energy used by traditional computers. They also participate in initiatives like the Climate Change AI (CCAI) consortium, which promotes the use of AI for climate solutions.
- Microsoft: Launching initiatives like AI for Earth to address environmental challenges
By choosing tech companies committed to sustainable practices and supporting research into energy-efficient AI solutions, we can ensure a future where AI progresses without compromising our planet.
The Future of Energy-Efficient AI
As we look ahead, several emerging trends promise to further reduce AI’s energy footprint:
- AI for Energy Management: Using AI itself to optimize energy consumption across various sectors
- Advancements in AI Hardware: Development of neuromorphic and optical computing technologies
- Green AI Practices: Industry-wide adoption of sustainable development and deployment practices
Conclusion: Balancing Progress and Sustainability
The future of AI hinges on our ability to balance technological advancement with environmental responsibility. By addressing energy constraints through innovative solutions, we can ensure that AI continues to progress without compromising our planet’s health.
As consumers and professionals in the tech industry, we play a crucial role in shaping this future. By supporting companies committed to sustainable AI practices and staying informed about energy-efficient technologies, we can contribute to a more sustainable AI ecosystem.
Stay at the forefront of sustainable AI development by subscribing to our Arcane AI Weekly Newsletter. Each week, we bring you cutting-edge insights, breakthrough technologies, and thought-provoking analyses on the intersection of AI and sustainability
Here are some resources to stay informed about sustainable AI advancements:
- The Partnership on AI (PAI): https://partnershiponai.org/
- The AI for Good website: https://aiforgood.itu.int/