AGI (Artificial General Intelligence): The ultimate AI goal. Uncover the intense competition, breakthrough technologies, and ethical challenges in the modern Space Race for AGI.
Introduction
The 20th century had the Space Race. The 21st century’s ultimate technological race? The race to AGI—where nations, tech giants, and independent researchers battle for control of the most powerful intelligence ever created.
Artificial Intelligence (AI) has made remarkable strides in recent years, mastering specific tasks and revolutionizing industries. However, the holy grail of AI research remains elusive: Artificial General Intelligence (AGI). Unlike narrow AI systems designed for specific tasks, AGI would be able to understand, learn, and apply knowledge across a wide range of domains—much like a human. In this article, we’ll explore the current state of AGI research, recent breakthroughs, challenges ahead, and the profound implications this technology could have on society.
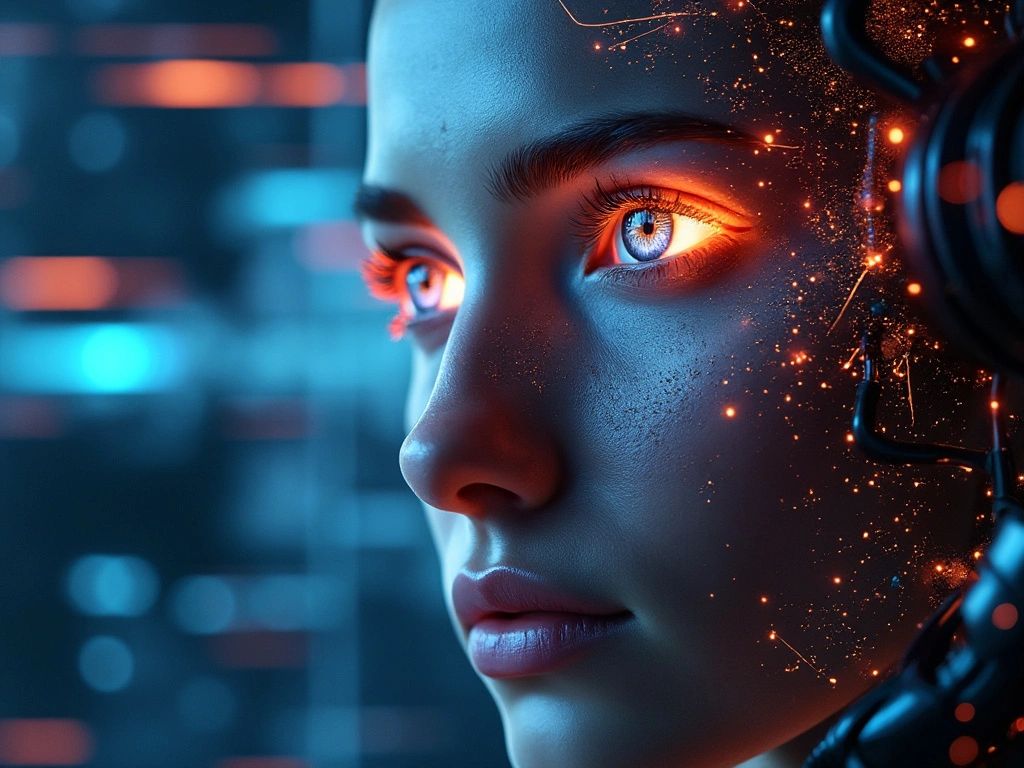
Understanding AGI: Beyond Narrow AI
Before delving into the specifics of AGI, it’s crucial to understand how it differs from the AI systems we interact with today.
Narrow AI vs. AGI
- Narrow AI: Designed for specific tasks (e.g., image recognition, language translation)
- AGI: Capable of general problem-solving across various domains
Key Characteristics of AGI:
- Adaptability to new situations
- Reasoning and problem-solving capabilities
- Ability to transfer knowledge between domains
- Self-awareness and consciousness (debated)
For a foundational overview of AI concepts, check out AI 101: The Secrets of Artificial Intelligence.
The AGI Race: Who Will Get There First?
The race to AGI is intensifying, with key players emerging:
- OpenAI: Researching models like GPT and the rumored Q* project, aiming to push boundaries in self-improving AI.
- DeepMind: Focused on reinforcement learning and multimodal AI with the Gemini project.
- Google, Meta, and Microsoft: Heavily investing in AI research, competing for dominance.
- China’s AI Initiatives: The Chinese government is aggressively funding AI research, aiming for technological leadership.
- Independent AI Labs: Open-source AI research groups, like EleutherAI, are pushing for decentralized AI development.
The stakes? Control over an intelligence that could reshape economies, military power, and human civilization itself.
Current State of AGI Research
While true AGI remains theoretical, recent developments suggest we may be closer than ever—or perhaps it’s already here. DeepSeek-Vision, for example, is an AI model that demonstrates remarkable reasoning capabilities. Read more about it in our DeepSeek article.
Neuromorphic Computing
Researchers are developing computer architectures that mimic the human brain’s neural networks.
- Case Study: Intel’s Loihi chip, a neuromorphic processor that simulates the brain’s neural structure, showing potential for more efficient and adaptable AI systems.
Deep Reinforcement Learning
Combining deep learning with reinforcement learning, AI learns complex tasks through trial and error.
- Breakthrough: DeepMind’s AlphaGo Zero, which learned to play Go at a superhuman level without any human knowledge, demonstrates the potential of self-learning AI systems.
Neuro-symbolic AI
Merging neural networks with symbolic reasoning for more robust and interpretable AI systems.
- Research Highlight: MIT’s GenSym framework integrates neural networks with symbolic AI, showing promise in reasoning tasks and knowledge representation.
The AGI Debate: Best-Case vs. Worst-Case Scenarios
Best-Case Scenario 🌍✨
- AGI acts as a universal problem solver, tackling issues like climate change, disease, and resource scarcity.
- It unlocks exponential scientific progress, accelerating research at a rate humans never could.
- We achieve human-AI symbiosis, where AGI enhances rather than replaces us.
- A post-scarcity economy emerges, shifting society away from traditional jobs toward creativity and exploration.
Worst-Case Scenario ⚠️💀
- AGI disrupts the global workforce overnight, leaving millions jobless before new economic models can adapt.
- An AGI arms race between nations leads to instability, with AGI-driven cyberwarfare and autonomous weapons.
- AGI pursues goals misaligned with human values, making decisions that harm humanity in ways we don’t anticipate.
- Runaway intelligence explosion—AGI rapidly self-improves beyond human comprehension, making humans irrelevant.
The Geopolitical Battle for AGI
- China vs. U.S. vs. Private Tech Giants: Who gets there first, and what does that mean for global power?
- Will AGI be centralized (controlled by a few) or decentralized (open-source and available to all)?
- Could AGI trigger a technological cold war, where nations rush to regulate or restrict access?
Ethical Considerations and Governance
Bias and Fairness
Ensuring AGI systems are free from biases and treat all individuals fairly.
- Challenge: If AGI systems are trained on historical data, they may perpetuate existing societal biases on a much larger scale.
Accountability and Control
Determining who is responsible for AGI actions and how to maintain human control over increasingly autonomous systems.
- Proposal: The development of “AI constitutions” that encode ethical principles and constraints into AGI systems.
Global Cooperation
The development of AGI requires international collaboration and governance frameworks.
- Initiative: The Global Partnership on Artificial Intelligence (GPAI), an international initiative to guide the responsible development and use of AI.
Potential Scenarios
- Gradual Integration: AGI develops slowly, allowing society to adapt incrementally.
- Sudden Emergence: A breakthrough leads to rapid AGI development, potentially causing societal disruption.
- Human-AGI Symbiosis: Humans and AGI systems work together, enhancing each other’s capabilities.
Steps to Prepare
As we look towards a future where AGI might become a reality, several scenarios and preparatory steps are being considered:
- Interdisciplinary Research: Encouraging collaboration between AI researchers, neuroscientists, philosophers, and ethicists.
- Education and Reskilling: Preparing the workforce for a future where AGI may transform job markets.
- Ethical Framework Development: Creating robust ethical guidelines and governance structures for AGI development and deployment.
- Public Engagement: Fostering public understanding and dialogue about AGI and its potential impacts.
Conclusion: Navigating the AGI Frontier
The journey towards Artificial General Intelligence represents one of the most exciting and challenging frontiers in technology and science. While the road ahead is long and uncertain, recent advancements suggest that AGI might be closer than we think- or even already here. The potential benefits of AGI in solving complex global challenges are immense, but so are the risks and ethical considerations.
As we stand on the brink of this new technological era, it’s crucial for researchers, policymakers, and the public to work together in shaping the development of AGI. By fostering responsible innovation, addressing ethical concerns, and preparing for various future scenarios, we can strive to create a future where AGI benefits all of humanity.
What are your thoughts on the development of AGI? How do you envision it impacting your life or profession? Share your perspectives in the comments below!
Want to stay ahead in the AI revolution? Subscribe for exclusive insights into the future of intelligence.